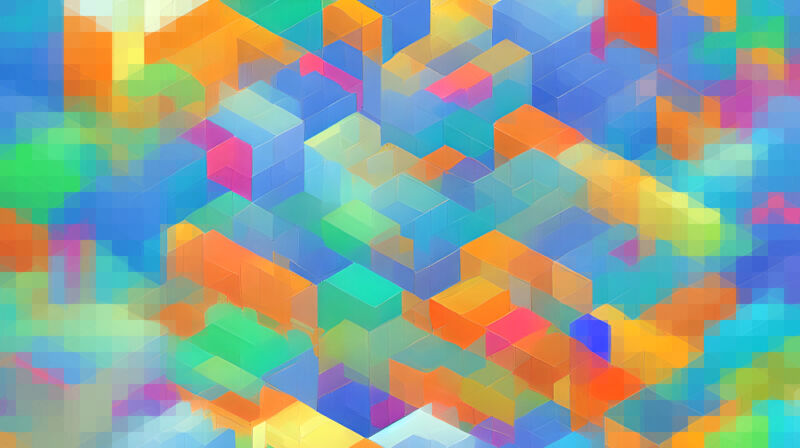
Enlarge / These jagged, colorful blocks are precisely what the conception of representation compression looks like.
Benj Edwards / Ars Technica
Last week, Swiss bundle technologist Matthias Bühlmann discovered that the fashionable representation synthesis exemplary Stable Diffusion could compress existing bitmapped images with less ocular artifacts than JPEG oregon WebP astatine precocious compression ratios, though determination are important caveats.
Stable Diffusion is an AI representation synthesis model that typically generates images based connected substance descriptions (called "prompts"). The AI exemplary learned this quality by studying millions of images pulled from the Internet. During the grooming process, the exemplary makes statistical associations betwixt images and related words, making a overmuch smaller practice of cardinal accusation astir each representation and storing them arsenic "weights," which are mathematical values that correspond what the AI representation exemplary knows, truthful to speak.
When Stable Diffusion analyzes and "compresses" images into value form, they reside successful what researchers telephone "latent space," which is simply a mode of saying that they beryllium arsenic a benignant of fuzzy imaginable that tin beryllium realized into images erstwhile they're decoded. With Stable Diffusion 1.4, the weights record is astir 4GB, but it represents cognition astir hundreds of millions of images.
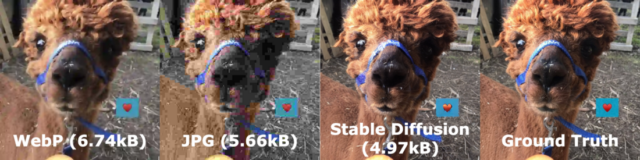
Enlarge / Examples of utilizing Stable Diffusion to compress images.
While astir radical usage Stable Diffusion with substance prompts, Bühlmann chopped retired the substance encoder and alternatively forced his images done Stable Diffusion's representation encoder process, which takes a low-precision 512×512 representation and turns it into a higher-precision 64×64 latent abstraction representation. At this point, the representation exists astatine a overmuch smaller information size than the original, but it tin inactive beryllium expanded (decoded) backmost into a 512×512 representation with reasonably bully results.
While moving tests, Bühlmann recovered that images compressed with Stable Diffusion looked subjectively amended astatine higher compression ratios (smaller record size) than JPEG oregon WebP. In 1 example, helium shows a photograph of a candy store that is compressed down to 5.68KB utilizing JPEG, 5.71KB utilizing WebP, and 4.98KB utilizing Stable Diffusion. The Stable Diffusion representation appears to person much resolved details and less evident compression artifacts than those compressed successful the different formats.
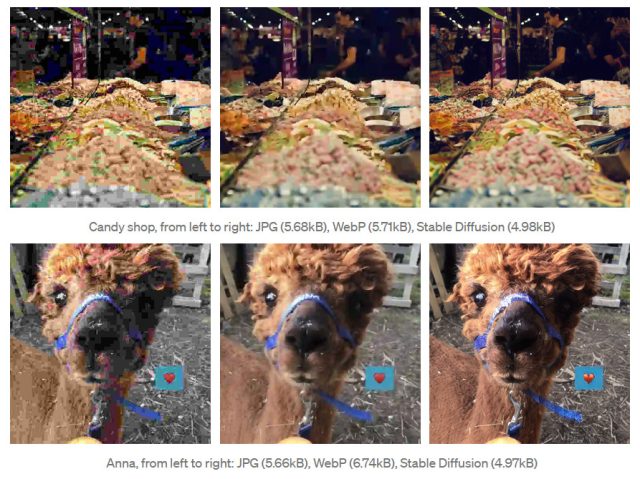
Enlarge / Experimental examples of utilizing Stable Diffusion to compress images. SD results are connected the acold right.
Bühlmann's method presently comes with important limitations, however: It's not bully with faces oregon text, and successful immoderate cases, it tin really hallucinate elaborate features successful the decoded representation that were not contiguous successful the root image. (You astir apt don't privation your representation compressor inventing details successful an representation that don't exist.) Also, decoding requires the 4GB Stable Diffusion weights record and other decoding time.
While this usage of Stable Diffusion is unconventional and much of a amusive hack than a applicable solution, it could perchance constituent to a caller aboriginal usage of representation synthesis models. Bühlmann's codification tin beryllium found connected Google Colab, and you'll find much method details astir his experimentation successful his post connected Towards AI.